Computer Archecture
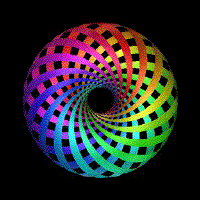
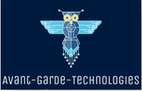
Future Computer infra-structure
Avant-Garde-Technologies Corporation (referred to as the Company or A-G-T) has 31 very large data center that are located in various different geographical areas though out the world that are over 100x100 meters. Solar power has been deployed in all of these data center that has significantly reduced the energy operating expenses. These data center are use to provide computer resources for the Company and its clients. These data center are deployed to provide a hybrid cloud and in-house computer resources. The vonNeumann architecture is used throughout these data centers for business and super Computing.
The Company is building 5 new 100x100 meters size data centers that will use Neuromorphic computer architecture for Artificial Intelligence (AI) and Deep Machine Learning (DML) that are necessary for learning applications that use visual, data and voice. Much of the AI applications rely upon visual data that requires enormous computer power. The Neuromorphic Architecture is well suited for learning from visual data applications, such as full self driving vehicles.
The Company also is developing software for Quantum Computer applications. It uses Quantum Computers developed by other companies for science and engineering applications. These applications frequently has AI-DMP embedded in the processes. It is rapidly approaching the time when Quantum Computing will be used with AI-DML to make significant advances in technologies, such as energy and biotech. The company currently uses AI-DML with Quantum Computing to model chemical reactions found in genetic engineering, proteins structure and for the Lithium Fluoride Thorium Reactors (LFTR) business segments. Because this type of computer technology is very new, it is expected to offer significant advances in these and other technologies.
Mozart - Symphony 40
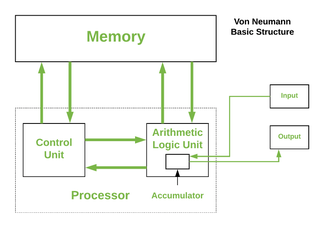
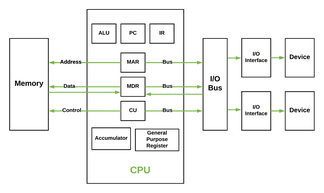
- Input/Output Devices – Program or data is read into main memory from the input device or secondary storage under the control of CPU input instruction. Output devices are used to output the information from a computer. If some results are evaluated by computer and it is stored in the computer, then with the help of output devices, we can present them to the user.
- Buses – Data is transmitted from one part of a computer to another, connecting all major internal components to the CPU and memory, by the means of Buses. Types:
- Data Bus: It carries data among the memory unit, the I/O devices, and the processor.
- Address Bus: It carries the address of data (not the actual data) between memory and processor.
- Control Bus: It carries control commands from the CPU (and status signals from other devices) in order to control and coordinate all the activities within the computer.
VonNeumann Architecture
The modern computers are based on a stored-program concept introduced by John Von Neumann. In this stored-program concept, programs and data are stored in a separate storage unit called memories and are treated the same. This novel idea meant that a computer built with this architecture would be much easier to reprogram.
The basic structure is also known as IAS computer and is having three basic units:
- The Central Processing Unit (CPU)
- The Main Memory Unit
- The Input/Output Device
Details
- Control Unit –
A control unit (CU) handles all processor control signals. It directs all input and output flow, fetches code for instructions, and controls how data moves around the system. - Arithmetic and Logic Unit (ALU) –
The arithmetic logic unit is that part of the CPU that handles all the calculations the CPU may need, e.g. Addition, Subtraction, Comparisons. It performs Logical Operations, Bit Shifting Operations, and Arithmetic operations.
Basic CPU structure, illustrating ALU
- Main Memory Unit (Registers) –
- Accumulator: Stores the results of calculations made by ALU.
- Program Counter (PC): Keeps track of the memory location of the next instructions to be dealt with. The PC then passes this next address to Memory Address Register (MAR).
- Memory Address Register (MAR): It stores the memory locations of instructions that need to be fetched from memory or stored into memory.
- Memory Data Register (MDR): It stores instructions fetched from memory or any data that is to be transferred to, and stored in, memory.
- Current Instruction Register (CIR): It stores the most recently fetched instructions while it is waiting to be coded and executed.
- Instruction Buffer Register (IBR): The instruction that is not to be executed immediately is placed in the instruction buffer register IBR.
Von Neumann bottleneck –
Whatever we do to enhance performance, we cannot get away from the fact that instructions can only be done one at a time and can only be carried out sequentially. Both of these factors hold back the competence of the CPU. This is commonly referred to as the ‘Von Neumann bottleneck’. We can provide a Von Neumann processor with more cache, more RAM, or faster components but if original gains are to be made in CPU performance then an influential inspection needs to take place of CPU configuration.
This architecture is very important and is used in our PCs and even in Super Computers.
Born To Survive - Troy Cassar-Daley
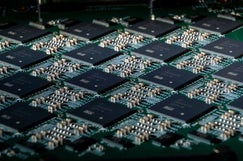
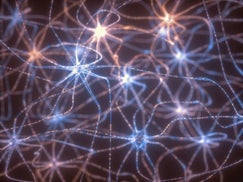
Bryan Nguyen - Pure Imagination
NeuroMorphic Architecture
Neuromorphic computing adds abilities to think creatively, recognize things they’ve never seen, and react accordingly to machines. Unlike AIs, the human brain is fascinating at understanding cause and effect and adapts to changes swiftly. However, even the slightest change in their environment renders AI models trained with traditional machine learning methods inoperable. Neuromorphic computing aims to overcome these challenges with brain-inspired computing methods.
Neuromorphic computing constructs spiking neural networks. Spikes from individual electronic neurons activate other neurons down a cascading chain, mimicking the physics of the human brain and nervous system. It works similar to how the brain sends and receives signals from neurons that spark computing. Neuromorphic chips compute more flexibly and broadly than conventional systems, which orchestrate computations in binary. Spiking neurons work without any specified pattern.
Neuromorphic computing achieves this brain-like performance and efficiency by constructing artificial neural networks out of “neurons” and “synapses.” Analog circuitry is used to connect these artificial neurons and synapses. They can modulate the amount of electricity flowing between those nodes, replicating natural brain signals’ various degrees of intensity.
These are spiking neural networks (SNN) in the brain, which can detect these distinct analog signal changes and aren’t present in conventional neural networks that employ less-nuanced digital signals.
Neuromorphic technology also envisions a new chip architecture that mixes memory and processing on each neuron rather than having distinct areas for one or the other.
Traditional chip designs based on the von Neumann architecture usually include a distinct memory unit, core processing unit (CPU), and data paths. The information must be transferred between various components as the computer completes a task, implying that data must travel back and forth numerous times. The von Neumann bottleneck is a limitation in time and energy efficiency when data transport across multiple components causes bottlenecks.
Neuromorphic computing provides a better way to handle massive amounts of data. It enables chips to be simultaneously very powerful and efficient. Depending on the situation, each neuron can perform processing or memory tasks.
Researchers are also working on alternate ways to model the brain’s synapse by utilizing quantum dots and graphene and memristive technologies, such as phase-change memory, resistive RAM, spin-transfer torque magnetic RAM, and conductive bridge RAM.
Traditional neural network and machine learning computations are well suited to current algorithms. They tend to prioritize performance or power, frequently resulting in one at the expense of the other. In contrast, neuromorphic systems deliver both rapid computing and low energy consumption.
Neuromorphic computing has two primary goals. The first is to construct a cognition machine that learns, retains data, and makes logical conclusions like humans. The second goal is to discover more about how the human brain works
Neuromorphic chips can perform many tasks simultaneously. Its event-driven working principle enables it to adapt to changing terms and conditions. These systems, which have computing capabilities such as generalization, are very flexible and robust. It’s highly fault-tolerant. Data is preserved redundantly, and even minor failures do not prevent overall performance. It can also solve complex issues and adapt to new settings swiftly.
Shubert - Symphony 8
Neuromorphic computing use cases
Edge computing devices like smartphones currently have to hand off processing to a cloud-based system, which processes the query and transmits the answer to the device for compute-intensive activities. That query wouldn’t have to be shunted back and forth with neuromorphic systems; it could be handled right on the device. The most important motivator of neuromorphic computing is the hope it provides for the future of AI.
AI systems are, by nature, highly rule-based; they’re trained on data until they can generate a particular outcome. On the other hand, our mind is much more natural to ambiguity and adaptability.
Researchers’ objective is to make the next generation of AI systems capable of dealing with more brain-like challenges. Constraint satisfaction is one of them and implies that machines must discover the best solution to a problem with many restrictions.
Neuromorphic chips are more comfortable with issues like probabilistic computing, where machines handle noisy and uncertain data. Other concepts, such as causality and non-linear thinking, are still in their infancy in neuromorphic computing systems but will mature once they become more widespread.
Neuromorphic computer systems available now
Many neuromorphic systems have been developed and utilized by academics, startups, and the most prominent players in the technology world.
Intel’s neuromorphic chip Loihi has 130 million synapses and 131,000 neurons. It was designed for spiking neural networks. Scientists use Intel Loihi chips to develop artificial skin and powered prosthetic limbs. Intel Labs’ second-generation neuromorphic research chip, codenamed Loihi 2, and Lava, an open-source software framework are also announced.
IBM’s neuromorphic system TrueNorth was unveiled in 2014, with 64 million neurons and 16 billion synapses. IBM recently announced a collaboration with the US Air Force Research Laboratory to develop a “neuromorphic supercomputer” known as Blue Raven. While the technology is still being developed, one use may be to develop smarter, lighter, and less energy-demanding drones.
The Human Brain Project (HBP), a 10-year project that began in 2013 and is funded by the European Union, was established to further understand the brain through six areas of study, including neuromorphic computing. The HBP has inspired two major neuromorphic projects from universities, SpiNNaker and BrainScaleS. In 2018, a million-core SpiNNaker system was introduced, becoming the world’s largest neuromorphic supercomputer at the time; The University of Manchester aims to scale it up to model one million neurons in the future.
The examples from IBM and Intel focus on computational performance. In contrast, the examples from the universities use neuromorphic computers as a tool for learning about the human brain. Both methods are necessary for neuromorphic computing since both types of information are required to advance AI.
Strauss - Thus Spoke Zarathustra
Intel Loihi neuromorphic chip architecture
Behind the Loihi chip architecture for neuromorphic computing lies the idea of ​​bringing computing power from the cloud to the edge. The second-generation Loihi neuromorphic research chip was introduced in April 2021, along with Lava, an open-source software architecture for applications inspired by the human brain. Neuromorphic computing adapts key features of neural architectures found in nature to create a new computer architecture model. Loihi was developed as a guide to the chips that would power these computers and Lava to the applications that would run on them.
According to the research paper Advancing Neuromorphic Computing with Loihi, the most striking feature of neuromorphic technology is its ability to mimic how the biological brain evolves to solve the challenges of interacting with dynamic and often unpredictable real-world environments. Neuromorphic chips that can mimic advanced biological systems include neurons, synapses for interneuron connections, and dendrites, allowing neurons to receive messages from more than one neuron.
A Loihi 2 chip consists of microprocessor cores and 128 fully asynchronous neuron cores interconnected by a network-on-chip (NoC). All communication between neuron nuclei optimized for neuromorphic workloads takes place in the form of impulse messages that mimic neural networks in a biological brain.
Instead of copying the human brain directly, neuromorphic computing diverges in different ways by being inspired by it; for example, in the Loihi chip, part of the chip functions as the neuron’s nucleus to model biological neuron behavior. In this model, a piece of code describes the neuron. On the other hand, there are neuromorphic systems in which biological synapses and dendrites are created using asynchronous digital complementary metal-oxide-semiconductor (CMOS) technology.
Memristor
Memristor, the core electronic component in neuromorphic chips, has been demonstrated to function similarly to brain synapses because it has plasticity similar to that of the brain. It is utilized to make artificial structures that mimic the brain’s abilities to process and memorize data. Until recently, the only three essential passive electrical components were capacitors, resistors, and inductors. The memristor created a stir when it was first discovered because it functioned as an integration of all three previously described types of passive components.
A memristor is a passive component that keeps a connection between the time integrals of current and voltage across a two-terminal element. As a result, the resistance of a memristor changes according to the memristance function of a device, allowing for access to memory via small read charges. This electrical component can remember its past states without being powered on. Although no energy flows through them, membrane memristors exhibit the ability to retain their previous states. They may also be used as memory and processing units in tandem.
Leon Chua first discovered the memristor in 1971 in theoretical form. In 2008, a research team at HP’s labs developed the first memristor from a thin film of Titanium Oxide. Since then, many other materials have been tested by various companies for the development of memristors.
The time it takes for data from the memory of a device to reach the processing unit is known as the von Neumann bottleneck. The processing unit must wait for the data required to perform computations. Because computations occur in the memory, neuromorphic chips do not experience this. Like how the brain works, memristors, which form the basis of neuromorphic chips, can be used for memory and calculation functions. They are composed of the first inorganic neurons, as we mentioned above.
Spiking neural networks (SNN) and artificial intelligence
The first generation of artificial intelligence was rule-based imitation logic, used to arrive at logical conclusions in a specific and restricted context. This tool is ideal for monitoring or optimizing a process. The focus was on artificial intelligence’s perception and detecting abilities. Deep Neural Networks have already been introduced into the application using conventional approaches such as SRAM or Flash-based. They mimic the parallelism and efficiency of the brain. Innovative DNN solutions may help to lower energy consumption for edge applications.
The next generation of artificial intelligence, which is already here and awaits our attention, extends these capabilities and fuses with human intellect, such as autonomous adaptation and the capacity to comprehend. This shift is critical to overcoming the current AI limitations caused by machine learning and inference. This is because such judgments, often uncontextualized and literal, are based on deterministic and literal interpretations of events that frequently lack context.
The next generation of AI must be able to respond to new circumstances and abstractions for it to automate common human activities. Spiking Neural Networks (SNN) also aims to replicate the temporal aspect of neurons and synapses functionality. This allows for more energy efficiency and flexibility.
The ability to adapt to a rapidly changing environment is one of the most challenging aspects of human intelligence. One of neuromorphic computing’s most intriguing problems is the capacity to learn from unstructured stimuli while being as energy-efficient as the human brain. The computer building blocks within neuromorphic computing systems are comparable to human neurons’ logic. Spiking Neural Network (SNN) is a new way of arranging these components to mimic human neural networks.
The Spiking Neural Network (SNN) is a type of artificial intelligence that creates outputs based on the responses given by its various neurons. Every neuron in the network may be triggered separately. Each neuron transmits pulsating signals to other neurons in the system, causing them to change their electrical state immediately. SNNs mimic natural learning processes by dynamically mapping synapses between artificial neurons in response to stimuli by interpreting this data within its signals and time.
Don't Worry - Marty Robbins
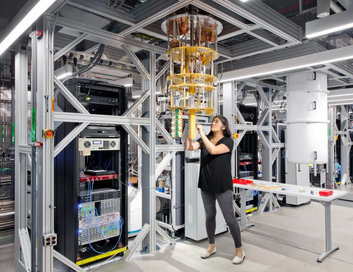
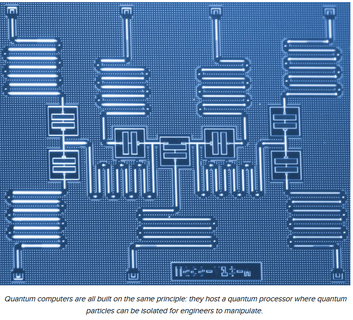
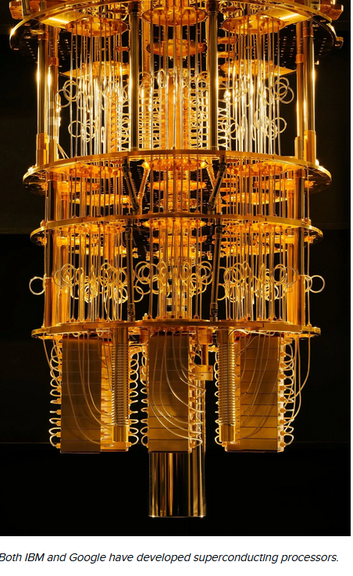
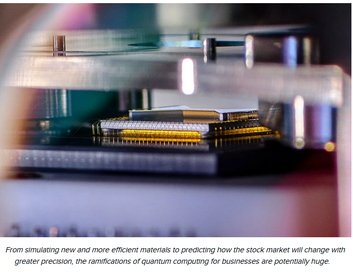
Rose Garden - Lynn Anderson
Quantum Computer
Quantum computing is a type of computation that harnesses the collective properties of quantum states, such as superposition, interference, and entanglement, to perform calculations. The devices that perform quantum computations are known as quantum computers.
Quantum computing exploits the puzzling behavior that scientists have been observing for decades in nature's smallest particles – think atoms, photons or electrons. At this scale, the classical laws of physics ceases to apply, and instead we shift to quantum rules.
While researchers don't understand everything about the quantum world, what they do know is that quantum particles hold immense potential, in particular to hold and process large amounts of information. Successfully bringing those particles under control in a quantum computer could trigger an explosion of compute power that would phenomenally advance innovation in many fields that require complex calculations, like drug discovery, climate modelling, financial optimization or logistics.
As Bob Sutor, chief quantum exponent at IBM, puts it: "Quantum computing is our way of emulating nature to solve extraordinarily difficult problems and make them tractable," he tells ZDNet.
What is a quantum computer?
Quantum computers come in various shapes and forms, but they are all built on the same principle: they host a quantum processor where quantum particles can be isolated for engineers to manipulate.
The nature of those quantum particles, as well as the method employed to control them, varies from one quantum computing approach to another. Some methods require the processor to be cooled down to freezing temperatures, others to play with quantum particles using lasers – but share the goal of finding out how to best exploit the value of quantum physics.
What's the difference between a quantum computer and a classical computer?
The systems we have been using since the 1940s in various shapes and forms – laptops, smartphones, cloud servers, supercomputers – are known as classical computers. Those are based on bits, a unit of information that powers every computation that happens in the device.
In a classical computer, each bit can take on either a value of one or zero to represent and transmit the information that is used to carry out computations. Using bits, developers can write programs, which are sets of instructions that are read and executed by the computer.
Classical computers have been indispensable tools in the past few decades, but the inflexibility of bits is limiting. As an analogy, if tasked with looking for a needle in a haystack, a classical computer would have to be programmed to look through every single piece of hay straw until it reached the needle.
There are still many large problems, therefore, that classical devices can't solve. "There are calculations that could be done on a classical system, but they might take millions of years or use more computer memory that exists in total on Earth," says Sutor. "These problems are intractable today."
How do quantum computers improve on classical devices?
At the heart of any quantum computer are qubits, also known as quantum bits, and which can loosely be compared to the bits that process information in classical computers.
Qubits, however, have very different properties to bits, because they are made of the quantum particles found in nature – those same particles that have been obsessing scientists for many years.
One of the properties of quantum particles that is most useful for quantum computing is known as superposition, which allows quantum particles to exist in several states at the same time. The best way to imagine superposition is to compare it to tossing a coin: instead of being heads or tails, quantum particles are the coin while it is still spinning.
By controlling quantum particles, researchers can load them with data to create qubits – and thanks to superposition, a single qubit doesn't have to be either a one or a zero, but can be both at the same time. In other words, while a classical bit can only be heads or tails, a qubit can be, at once, heads and tails.
This means that, when asked to solve a problem, a quantum computer can use qubits to run several calculations at once to find an answer, exploring many different avenues in parallel.
So in the needle-in-a-haystack scenario about, unlike a classical machine, a quantum computer could in principle browse through all hay straws at the same time, finding the needle in a matter of seconds rather than looking for years – even centuries – before it found what it was searching for.
What's more: qubits can be physically linked together thanks to another quantum property called entanglement, meaning that with every qubit that is added to a system, the device's capabilities increase exponentially – where adding more bits only generates linear improvement.
Every time we use another qubit in a quantum computer, we double the amount of information and processing ability available for solving problems. So by the time we get to 275 qubits, we can compute with more pieces of information than there are atoms in the observable universe. And the compression of computing time that this could generate could have big implications in many use cases.
Why is quantum computing so important?
"There are a number of cases where time is money. Being able to do things more quickly will have a material impact in business," Scott Buchholz, managing director at Deloitte Consulting, tells ZDNet.
The gains in time that researchers are anticipating as a result of quantum computing are not of the order of hours or even days. We're rather talking about potentially being capable of calculating, in just a few minutes, the answer to problems that today's most powerful supercomputers couldn't resolve in thousands of years, ranging from modelling hurricanes all the way to cracking the cryptography keys protecting the most sensitive government secrets.
And businesses have a lot to gain, too. According to recent research by Boston Consulting Group (BCG), the advances that quantum computing will enable could create value of up to $850 billion in the next 15 to 30 years,
What is a quantum computer used for?
Programmers write problems in the form of algorithms for classical computers to resolve – and similarly, quantum computers will carry out calculations based on quantum algorithms. Researchers have already identified that some quantum algorithms would be particularly suited to the enhanced capabilities of quantum computers.
For example, quantum systems could tackle optimization algorithms, which help identify the best solution among many feasible options, and could be applied in a wide range of scenarios ranging from supply chain administration to traffic management. ExxonMobil and IBM, for instance, are working together to find quantum algorithms that could one day manage the 50,000 merchant ships crossing the oceans each day to deliver goods, to reduce the distance and time traveled by fleets.
Quantum simulation algorithms are also expected to deliver unprecedented results, as qubits enable researchers to handle the simulation and prediction of complex interactions between molecules in larger systems, which could lead to faster breakthroughs in fields like materials science and drug discovery.
With quantum computers capable of handling and processing much larger datasets, AI and machine-learning applications are set to benefit hugely, with faster training times and more capable algorithms. And researchers have also demonstrated that quantum algorithms have the potential to crack traditional cryptography keys, which for now are too mathematically difficult for classical computers to break.
Blondie - One Way or Another
What are the different types of quantum computers?
To create qubits, which are the building blocks of quantum computers, scientists have to find and manipulate the smallest particles of nature – tiny parts of the universe that can be found thanks to different mediums. This is why there are currently many types of quantum processors being developed by a range of companies.
One of the most advanced approaches consists of using superconducting qubits, which are made of electrons, and come in the form of the familiar chandelier-like quantum computers. Both IBM and Google have developed superconducting processors.
Another approach that is gaining momentum is trapped ions, which Honeywell and IonQ are leading the way on, and in which qubits are housed in arrays of ions that are trapped in electric fields and then controlled with lasers.
Major companies like Xanadu and PsiQuantum, for their part, are investing in yet another method that relies on quantum particles of light, called photons, to encode data and create qubits. Qubits can also be created out of silicon spin qubits – which Intel is focusing on – but also cold atoms or even diamonds.
Quantum annealing, an approach that was chosen by D-Wave, is a different category of computing altogether. It doesn't rely on the same paradigm as other quantum processors, known as the gate model. Quantum annealing processors are much easier to control and operate, which is why D-Wave has already developed devices that can manipulate thousands of qubits, where virtually every other quantum hardware company is working with about 100 qubits or less. On the other hand, the annealing approach is only suitable for a specific set of optimization problems, which limits its capabilities.
Beethoven - Moonlight Sonata
What can you do with a quantum computer today?
Right now, with a mere 100 qubits being the state of the art, there is very little that can actually be done with quantum computers. For qubits to start carrying out meaningful calculations, they will have to be counted in the thousands, and even millions.
"While there is a tremendous amount of promise and excitement about what quantum computers can do one day, I think what they can do today is relatively underwhelming," says Buchholz.
Increasing the qubit count in gate-model processors, however, is incredibly challenging. This is because keeping the particles that make up qubits in their quantum state is difficult – a little bit like trying to keep a coin spinning without falling on one side or the other, except much harder.
Keeping qubits spinning requires isolating them from any environmental disturbance that might cause them to lose their quantum state. Google and IBM, for example, do this by placing their superconducting processors in temperatures that are colder than outer space, which in turn require sophisticated cryogenic technologies that are currently near-impossible to scale up.
In addition, the instability of qubits means that they are unreliable, and still likely to cause computation errors. This has given rise to a branch of quantum computing dedicated to developing error-correction methods.
Although research is advancing at pace, therefore, quantum computers are for now stuck in what is known as the NISQ era: noisy, intermediate-scale quantum computing – but the end-goal is to build a fault-tolerant, universal quantum computer.
As Buchholz explains, it is hard to tell when this is likely to happen. "I would guess we are a handful of years from production use cases, but the real challenge is that this is a little like trying to predict research breakthroughs," he says. "It's hard to put a timeline on genius."
What is quantum supremacy?
In 2019, Google claimed that its 54-qubit superconducting processor called Sycamore had achieved quantum supremacy – the point at which a quantum computer can solve a computational task that is impossible to run on a classical device in any realistic amount of time.
Google said that Sycamore has calculated, in only 200 seconds, the answer to a problem that would have taken the world's biggest supercomputers 10,000 years to complete.
More recently, researchers from the University of Science and Technology of China claimed a similar breakthrough, saying that their quantum processor had taken 200 seconds to achieve a task that would have taken 600 million years to complete with classical devices.
This is far from saying that either of those quantum computers are now capable of outstripping any classical computer at any task. In both cases, the devices were programmed to run very specific problems, with little usefulness aside from proving that they could compute the task significantly faster than classical systems.
Without a higher qubit count and better error correction, proving quantum supremacy for useful problems is still some way off.
What is the use of quantum computers now?
Organizations that are investing in quantum resources see this as the preparation stage: their scientists are doing the groundwork to be ready for the day that a universal and fault-tolerant quantum computer is ready.
In practice, this means that they are trying to discover the quantum algorithms that are most likely to show an advantage over classical algorithms once they can be run on large-scale quantum systems. To do so, researchers typically try to prove that quantum algorithms perform comparably to classical ones on very small use cases, and theorize that as quantum hardware improves, and the size of the problem can be grown, the quantum approach will inevitably show some significant speed-ups.
For example, scientists at Japanese steel manufacturer Nippon Steel recently came up with a quantum optimization algorithm that could compete against its classical counterpart for a small problem that was run on a 10-qubit quantum computer. In principle, this means that the same algorithm equipped with thousands or millions of error-corrected qubits could eventually optimize the company's entire supply chain, complete with the management of dozens of raw materials, processes and tight deadlines, generating huge cost savings.
The work that quantum scientists are carrying out for businesses is, therefore, highly experimental, and so far there are fewer than 100 quantum algorithms that have been shown to compete against their classical equivalents – which only points to how emergent the field still is.
Who is going to win the quantum computing race?
With most use cases requiring a fully error-corrected quantum computer, just who will deliver one first is the question on everyone's lips in the quantum industry, and it is impossible to know the exact answer.
All quantum hardware companies are keen to stress that their approach will be the first one to crack the quantum revolution, making it even harder to discern noise from reality. "The challenge at the moment is that it's like looking at a group of toddlers in a playground and trying to figure out which one of them is going to win the Nobel Prize," says Buchholz.
"I have seen the smartest people in the field say they're not really sure which one of these is the right answer. There are more than half a dozen different competing technologies and it's still not clear which one will wind up being the best, or if there will be a best one," he continues.
In general, experts agree that the technology will not reach its full potential until after 2030. The next five years, however, may start bringing some early use cases as error correction improves and qubit counts start reaching numbers that allow for small problems to be programmed.
IBM is one of the rare companies that has committed to a specific quantum roadmap, which defines the ultimate objective of realizing a million-qubit quantum computer. In the nearer term, Big Blue anticipates that it will release a 1,121-qubit system in 2023, which might mark the start of the first experimentations with real-world use cases.
What about quantum software?
Developing quantum hardware is a huge part of the challenge, and arguably the most significant bottleneck in the ecosystem. But even a universal fault-tolerant quantum computer would be of little use without the matching quantum software.
"Of course, none of these online facilities are much use without knowing how to 'speak' quantum," Andrew Fearnside, senior associate specializing in quantum technologies at intellectual property firm Mewburn Ellis, tells ZDNet.
Creating quantum algorithms is not as easy as taking a classical algorithm and adapting it to the quantum world. Quantum computing, rather, requires a brand-new programming paradigm that can only be run on a brand-new software stack.
Of course, some hardware providers also develop software tools, the most established of which is IBM's open-source quantum software development kit Qiskit. But on top of that, the quantum ecosystem is expanding to include companies dedicated exclusively to creating quantum software. Familiar names include Zapata, QC Ware or 1QBit, which all specialize in providing businesses with the tools to understand the language of quantum.
And increasingly, promising partnerships are forming to bring together different parts of the ecosystem. For example, the recent alliance between Honeywell, which is building trapped ions quantum computers, and quantum software company Cambridge Quantum Computing (CQC), has got analysts predicting that a new player could be taking a lead in the quantum race.
What is cloud quantum computing?
The complexity of building a quantum computer – think ultra-high vacuum chambers, cryogenic control systems and other exotic quantum instruments – means that the vast majority of quantum systems are currently firmly sitting in lab environments, rather than being sent out to customers' data centers.
To let users access the devices to start running their experiments, therefore, quantum companies have launched commercial quantum computing cloud services, making the technology accessible to a wider range of customers.
The four largest providers of public cloud computing services currently offer access to quantum computers on their platform. IBM and Google have both put their own quantum processors on the cloud, while Microsoft's Azure Quantum and AWS's Braket service let customers access computers from third-party quantum hardware providers.
Who is getting quantum-ready now?
Although not all businesses need to be preparing themselves to keep up with quantum-ready competitors, there are some industries where quantum algorithms are expected to generate huge value, and where leading companies are already getting ready.
Goldman Sachs and JP Morgan are two examples of financial behemoths investing in quantum computing. That's because in banking, quantum optimization algorithms could give a boost to portfolio optimization, by better picking which stocks to buy and sell for maximum return.
In pharmaceuticals, where the drug discovery process is on average a $2 billion, 10-year-long deal that largely relies on trial and error, quantum simulation algorithms are also expected to make waves. This is also the case in materials science: companies like OTI Lumionics, for example, are exploring the use of quantum computers to design more efficient OLED displays.
Leading automotive companies including Volkswagen and BMW are also keeping a close eye on the technology, which could impact the sector in various ways, ranging from designing more efficient batteries to optimizing the supply chain, through to better management of traffic and mobility. Volkswagen, for example, pioneered the use of a quantum algorithm that optimized bus routes in real time by dodging traffic bottlenecks.
As the technology matures, however, it is unlikely that quantum computing will be limited to a select few. Rather, analysts anticipate that virtually all industries have the potential to benefit from the computational speedup that qubits will unlock.
What does the quantum computing industry look like today?
The jury remains out on which technology will win the race, if any at all, but one thing is for certain: the quantum computing industry is developing fast, and investors are generously funding the ecosystem. Equity investments in quantum computing nearly tripled in 2020, and according to BCG, they are set to rise even more in 2021 to reach $800 million.
Government investment is even more significant: the US has unlocked $1.2 billion for quantum information science over the next five years, while the EU announced a €1 billion ($1.20 billion) quantum flagship. The UK also recently reached the £1 billion ($1.37 billion) budget milestone for quantum technologies, and while official numbers are not known in China, the government has made no secret of its desire to aggressively compete in the quantum race.
This has caused the quantum ecosystem to flourish over the past years, with new startups increasing from a handful in 2013 to nearly 200 in 2020. The appeal of quantum computing is also increasing among potential customers: according to analysis firm Gartner, while only 1% of companies were budgeting for quantum in 2018, 20% are expected to do so by 2023.
Will quantum computers replace our laptops?
Quantum computers are expected to be phenomenal at solving a certain class of problems, but that doesn't mean that they will be a better tool than classical computers for every single application. Particularly, quantum systems aren't a good fit for fundamental computations like arithmetic, or for executing commands.
"Quantum computers are great constraint optimizers, but that's not what you need to run Microsoft Excel or Office," says Buchholz. "That's what classical technology is for: for doing lots of maths, calculations and sequential operations."
In other words, there will always be a place for the way that we compute today. It is unlikely, for example, that you will be streaming a Netflix series on a quantum computer anytime soon. Rather, the two technologies will be used in conjunction, with quantum computers being called for only where they can dramatically accelerate a specific calculation.
How will we use quantum computers?
Buchholz predicts that, as classical and quantum computing start working alongside each other, access will look like a configuration option. Data scientists currently have a choice of using CPUs or GPUs when running their workloads, and it might be that quantum processing units (QPUs) join the list at some point. It will be up to researchers to decide which configuration to choose, based on the nature of their computation.
Although the precise way that users will access quantum computing in the future remains to be defined, one thing is certain: they are unlikely to be required to understand the fundamental laws of quantum computing in order to use the technology.
"People get confused because the way we lead into quantum computing is by talking about technical details," says Buchholz. "But you don't need to understand how your cellphone works to use it.
"People sometimes forget that when you log into a server somewhere, you have no idea what physical location the server is in or even if it exists physically at all anymore. The important question really becomes what it is going to look like to access it."
And as fascinating as qubits, superposition, entanglement and other quantum phenomena might be, for most of us this will come as welcome news.
Quantum computing is going to change the world
The world's biggest companies are now launching quantum computing programs, and governments are pouring money into quantum research. For systems that have yet prove useful, quantum computers are certainly garnering lots of attention.
The reason is that quantum computers, although still far from having reached maturity, are expected to eventually usher in a whole new era of computing -- one in which the hardware is no longer a constraint when resolving complex problems, meaning that some calculations that would take years or even centuries for classical systems to complete could be achieved in minutes.
From simulating new and more efficient materials to predicting how the stock market will change with greater precision, the ramifications for businesses are potentially huge. Here are eight quantum use cases that leading organisations are exploring right now, which could radically change the game across entire industries.
1. Discovering new drugs
The discovery of new drugs relies in part on a field of science known as molecular simulation, which consists of modelling the way that particles interact inside a molecule to try and create a configuration that's capable of fighting off a given disease.
Those interactions are incredibly complex and can assume many different shapes and forms, meaning that accurate prediction of the way that a molecule will behave based on its structure requires huge amounts of calculation.
Doing this manually is impossible, and the size of the problem is also too large for today's classical computers to take on. In fact, it's expected that modelling a molecule with only 70 atoms would take a classical computer up to 13 billion years.
This is why discovering new drugs takes so long: scientists mostly adopt a trial-and-error approach, in which they test thousands of molecules against a target disease in the hope that a successful match will eventually be found.
Quantum computers, however, have the potential to one day resolve the molecular simulation problem in minutes. The systems are designed to be able to carry out many calculations at the same time, meaning that they could seamlessly simulate all of the most complex interactions between particles that make up molecules, enabling scientists to rapidly identify candidates for successful drugs.
This would mean that life-saving drugs, which currently take an average 10 years to reach the market, could be designed faster -- and much more cost-efficiently.
Pharmaceutical companies are paying attention: earlier this year, healthcare giant Roche announced a partnership with Cambridge Quantum Computing (CQC) to support efforts in research tackling Alzheimer's disease.
And smaller companies are also taking interest in the technology. Synthetic biology start-up Menten AI, for example, has partnered with quantum annealing company D-Wave to explore how quantum algorithms could help design new proteins that could eventually be used as therapeutic drugs.
2. Creating better batteries
From powering cars to storing renewable energy, batteries are already supporting the transition to a greener economy, and their role is only set to grow. But they are far from perfect: their capacity is still limited, and so is their charging speed, which means that they are not always a suitable option.
One solution consists of searching for new materials with better properties to build batteries. This is another molecular simulation problem -- this time modelling the behaviour of molecules that could be potential candidates for new battery materials.
Similar to drug design, therefore, battery design is another data-heavy job that's better suited to a quantum computer than a classical device.
This is why German car manufacturer Daimler has now partnered with IBM to assess how quantum computers could help simulate the behaviour of sulphur molecules in different environments, with the end-goal of building lithium-sulphur batteries that are better-performing, longer-lasting and less expensive that today's lithium-ion ones.
3. Predicting the weather
Despite the vast amounts of compute power available from today's cutting-edge supercomputers, weather forecasts -- particularly longer-range ones -- can still be disappointingly inaccurate. This is because there are countless ways that a weather event might manifest itself, and classical devices are incapable of ingesting all of the data required for a precise prediction.
On the other hand, just as quantum computers could simulate all of the particle interactions going on within a molecule at the same time to predict its behaviour, so could they model how innumerable environmental factors all come together to create a major storm, a hurricane or a heatwave.
And because quantum computers would be able to analyse virtually all of the relevant data at once, they are likely to generate predictions that are much more accurate than current weather forecasts. This isn't only good for planning your next outdoor event: it could also help governments better prepare for natural disasters, as well as support climate-change research.
Research in this field is quieter, but partnerships are emerging to take a closer look at the potential of quantum computers. Last year, for instance, the European Centre for Medium-Range Weather Forecasts (ECMWF) launched a partnership with IT company Atos that included access to Atos's quantum computing simulator, in a bid to explore how quantum computing may impact weather and climate prediction in the future.
4. Picking stocks
JP Morgan, Goldman Sachs and Wells Fargo are all actively investigating the potential of quantum computers to improve the efficiency of banking operations -- a use case often put forward as one that could come with big financial rewards.
There are several ways that the technology could support the activities of banks, but one that's already showing promise is the application of quantum computing to a procedure known as Monte Carlo simulation.
The Monte Carlo operation consists of pricing financial assets based on how the price of related assets changes over time, meaning that it's necessary to account for the risk inherent in different options, stocks, currencies and commodities. The procedure essentially boils down to predicting how the market will evolve -- an exercise that becomes more accurate with larger amounts of relevant data.
Quantum computers' unprecedented computation abilities could speed up Monte Carlo calculations by up to 1,000 times, according to research carried out by Goldman Sachs together with quantum computing company QC Ware. In even more promising news, Goldman Sachs' quantum engineers have now tweaked their algorithms to be able to run the Monte Carlo simulation on quantum hardware that could be available in as little as five years' time.
5. Processing language
For decades, researchers have tried to teach classical computers how to associate meaning with words to try and make sense of entire sentences. This is a huge challenge given the nature of language, which functions as an interactive network: rather than being the 'sum' of the meaning of each individual word, a sentence often has to be interpreted as a whole. And that's before even trying to account for sarcasm, humour or connotation.
As a result, even state-of-the-art natural language processing (NLP) classical algorithms can still struggle to understand the meaning of basic sentences. But researchers are investigating whether quantum computers might be better suited to representing language as a network -- and, therefore, to processing it in a more intuitive way.
The field is known as quantum natural language processing (QNLP), and is a key focus of Cambridge Quantum Computing (CQC). The company has already experimentally shown that sentences can be parameterised on quantum circuits, where word meanings can be embedded according to the grammatical structure of the sentence. More recently, CQC released lambeq, a software toolkit for QNLP that can convert sentences into a quantum circuit.
6. Helping to solve the travelling salesman problem
A salesman is given a list of cities they need to visit, as well as the distance between each city, and has to come up with the route that will save the most travel time and cost the least money. As simple as it sounds, the 'travelling salesman problem' is one that many companies are faced with when trying to optimise their supply chains or delivery routes.
With every new city that is added to the salesman list, the number of possible routes multiplies. And at the scale of a multinational corporation, which is likely to be dealing with hundreds of destinations, a few thousand fleets and strict deadlines, the problem becomes much too large for a classical computer to resolve in any reasonable time.
Energy giant ExxonMobil, for example, has been trying to optimise the daily routing of merchant ships crossing the oceans -- that is, more than 50,000 ships carrying up to 200,000 containers each, to move goods with a total value of $14 trillion.
Some classical algorithms exist already to tackle the challenge. But given the huge number of possible routes to explore, the models inevitably have to resort to simplifications and approximations. ExxonMobil, therefore, teamed up with IBM to find out if quantum algorithms could do a better job.
Quantum computers' ability to take on several calculations at once means that they could run through all of the different routes in tandem, allowing them to discover the most optimal solution much faster than a classical computer, which would have to evaluate each option sequentially.
ExxonMobil's results seem promising: simulations suggest that IBM's quantum algorithms could provide better results than classical algorithms once the hardware has improved.
7. Reducing congestion
Optimising the timing of traffic signals in cities, so that they can adapt to the number of vehicles waiting or the time of day, could go a long way towards smoothing the flow of vehicles and avoiding congestion at busy intersections.
This is another problem that classical computers find hard: the more variables there are, the more possibilities have to be computed by the system before the best solution is found. But as with the travelling salesman problem, quantum computers could assess different scenarios at the same time, reaching the most optimal outcome a lot more rapidly.
Microsoft has been working on this use case together with Toyoto Tsusho and quantum computing startup Jij. The researchers have begun developing quantum-inspired algorithms in a simulated city environment, with the goal of reducing congestion. According to the experiment's latest results, the approach could bring down traffic waiting times by up to 20%.
8. Protecting sensitive data
Modern cryptography relies on keys that are generated by algorithms to encode data, meaning that only parties granted access to the key have the means to decrypt the message. The risk, therefore, is two-fold: hackers can either intercept the cryptography key to decipher the data, or they can use powerful computers to try and predict the key that has been generated by the algorithm.
This is because classical security algorithms are deterministic: a given input will always produce the same output, which means that with the right amount of compute power, a hacker can predict the result.
This approach requires extremely powerful computers, and isn't considered a near-term risk for cryptography. But hardware is improving, and security researchers are increasingly warning that more secure cryptography keys will be needed at some point in the future.
One way to strengthen the keys, therefore, is to make them entirely random and illogical -- in other words, impossible to guess mathematically.
And as it turns out, randomness is a fundamental part of quantum behaviour: the particles that make up a quantum processor, for instance, behave in completely unpredictable ways. This behaviour can, therefore, be used to determine cryptography keys that are impossible to reverse-engineer, even with the most powerful supercomputer.
Random number generation is an application of quantum computing that is already nearing commercialisation. UK-based startup Nu Quantum, for example, is finalizing a system that can measure the behavior of quantum particles to generate streams of random numbers that can then be used to build stronger cryptography keys.
Twisted Sister - We're Not Gonna Take
Photonic or Optical Computers
A type of computer in which the electronic circuits, which process data serially, are replaced by photonic circuits capable of parallel processing and thus of much greater speed and power. Where the standard electronic circuitry of a conventional computer utilizes electrons, the photonic computer uses photons for computation. These photons are generated by diodes, and/or lasers.
What is Optical Computing
When I say optical computing, what exactly comes across your mind. What is optical computing? Some of you might guess that it would be something related to light (because of the word optical!). Some of you may think about its computing speed and compare it you light speed. Don’t be in confusion, mate. Hypothetically, optical computing is computing using visible lights or infrared beams (IR) instead of electric current. You might wonder how it is possible. Don’t worry; just read ahead. When I am done, you will know all the things you need to know about it.
What Is Optical Computing?
By now, you have understood that it is something related to light. Optical computing is basically a new technological approach for making the computing process better using optics and related technologies. Optical computing (also photonic computing) uses photons in the computing process which give more bandwidth naturally than the electrons used in conventional computing.
To control electricity, the conventional computers that we use today use transistors and semiconductors. The optical computers of the future may use crystals and metamaterials to control light. It means that all the component of the computer should be light based.
The entirely optical computer isn’t possibly implemented yet; it might be in the near future. But, electro-optical hybrid computing is already implemented and it has been done successfully to do some basic operations.
Why Do We Need Optical Computers?
- With the growth of computing technology, the need for better computers which have better computing performance has increased significantly.
- There is a rapid growth of the internet at the present time.
- The speed of the network and system is limited by the electronic circuitry system.
- There is a physical limit of the tradition silicon-based
- Again, optical computing is very fast and need less energy.
Types of Optical Computers
The types of optical computers are not constant and universal rather controversial. There might be so many version of it.
- Optical analog: This type of optical computers includes 2-D Fourier transform, optical matrix-vector processors, etc.
- Optoelectronics: This type includes the feature of shortening the pulse delay by using optical interconnections in usual chips and other logic elements.
- Optical parallel digital computers: For, flexibility, along with digital electronics this type of optical computer uses the inherent parallelism which is a feature of optical devices.
- Optical neural computers: This type uses a special kind of computing process which doesn’t require any kind of ordinary programming. They usually have streams of I/O bits (Input Output bits).
How Does Optical Computing Work?
Electronic circuits are the basis of a conventional computer which is choreographed to correspond by switching one another on and off in a sequential way very carefully. Optical computing also follows a similar kind of principle but in a different manner. The calculations are performed by beams of photons instead of streams of electrons. These photons interact with one another and with guiding components such as lenses. The electrons, against a tide of resistance, have to flow through twists and turns of circuitry whereas, the photons have technically no mass, can travel at speed of light and draw less energy.
So, the working principle of optical computers is mainly photon based. They have photonic circuits and organics-compound components. No short-circuit is possible theoretically. They have no heat dissipation The speed in the photonic circuit is high (close to light).
Component of Optical Computer
The components can be varied depending on the type of optical computers.
- Vertical Cavity Surface Emitting Laser or VCSEL (a diode that emits light in a cylindrical beam)
- Smart Pixel Technology
- Wavelength Division Multiplexing or WDM (method of sending different types of wavelengths through same optical fiber)
- Photonic switches
- Spatial Light Modulator or SLM
- Optical memory/holographic storage
Application of Optical Computing
What actually can these optical computers do? What are its applications? Okay, optical computing has a number of significant applications.
- In Communication: Optical computing has a good number of applications in the communication process. They can be used in Wavelength Division Multiplexing, Optical Amplifiers, Storage Area Network, Fiber Channel topology, etc. Optical computing makes the communication process faster, more efficient and give better performance.
- In VLSI technology: VLSI is Very Large Scale Integration. For solving different types of hard problems, optical computing may play a big role by helping in the VLSI technology simplification.
- As expanders: It can be used as expander which gives high speed and high bandwidth product connections.
- In solving NP-hard problems: The problems which are unsolvable by conventional computers, might be solved by optical computers.
Why Optical Computing Is Better Than the Conventional Computing
The optical computing has many advantages over conventional silicon-based computing.
- The optical computing is very faster (10 to 100 to 1000) than today’s conventional computing.
- The optical storage is far better than today’s storage system which gives an optimized way to store data by taking less space.
- In optical computing, the searches through databases are very fast.
- In it, there is no short circuit. The light beams can pass each other without interacting and interfere with each other’s data.
- Unlike electric crossover, the optical crossover doesn’t need three-dimensional
- The optical computers have higher performance.
- They have higher parallelism
- They produce less heat and noise
- In optical computing, the communication process will be made optimized and will have less loss in it.
The can solve such problems which are currently unsolvable for the physical limits of silicon-based
Limitation/Drawbacks
In spite of having many advantages, optical computing has some limitations also. The optoelectronic computing is already implemented, but scientists aren’t able to build pure optical computers. Optical components and so their products are very expensive. The dust or imperfections may create scattering effects; due to this, unwanted interference pattern might be created. In optoelectronic computing, there is a basic speed limit because of conversion delays.
Optical computing may open many new possibilities in several fields. Researches are going on and the optical digital computers are yet to come. This might start the beginning of a new era of high performance and speed.
Future of Photonic computing
The future is optical. Photonic processors promise blazing fast calculation speeds with much lower power demands, and they could revolutionise machine learning.
Photonic computing is as the name suggests, a computer system that uses optical light pulses to form the basis of logic gates rather than electrical transistors. If it can be made to work in such a way that processors can be mass produced at a practical size it has the potential to revolutionise machine learning and other specific types of computing tasks. The emphasis being on the word if. However there are some intriguing sounding products close to coming to market that could changes things drastically.
The idea behind photonic computers is not a new one, with optical matrix multiplications first being demonstrated in the 1970s, however nobody has managed to solve many of the roadblocks to getting them to work on a practical level that can be integrated as easily as transistor based systems. Using photons is an obvious choice to help speed things up. After all all new homes in the UK are built with fibre to the home for a reason. Fibre optic cables are superior to aluminium or copper wires for the modern world of digital data communication. They can transmit more information faster, and over longer distances without signal degradation than metal wiring. However transmitting data from A to B is a whole different kettle of fish to putting such optical pipelines onto a chip fabrication that allows for matrix processing, even though some data centres already use optical cables for faster internal data transfer over short distances.
In an article for IEEE Spectrum, Ryan Hamerly puts forward the case for photonic chips along with proposals for solving the key issues, for which there are still many.
First and foremost is the way in which traditional processors work. Traditional transistor based chips are non-linear. Hamerly states “Nonlinearity is what lets transistors switch on and off, allowing them to be fashioned into logic gates. This switching is easy to accomplish with electronics, for which nonlinearities are a dime a dozen. But photons follow Maxwell’s equations, which are annoyingly linear, meaning that the output of an optical device is typically proportional to its inputs. The trick is to use the linearity of optical devices to do the one thing that deep learning relies on most: linear algebra.”
For his own solution, Hamerly proposes using an optical beam splitter that allows two perpendicular beams of light to be fired at it from opposite directions. Each beam of light is split by allowing half of the light to pass through to the other side of the beam splitter mirror, while the remaining light is bounced at 90 degrees from its origin.
The result is that this beam splitter designs allows for two inputs and two outputs, which in turn makes matrix multiplication possible, which is how a traditional computer performs its calculations. Hamerly states that to make this work two light beams are generated with electric-field intensifies that are proportional to the two numbers you wish to multiply, which he calls field intensities x and y. The two beams are fired into the beam splitter and combined in such a way that it produces two outputs.
Hamerly says, “In addition to the beam splitter, this analog multiplier requires two simple electronic components—photodetectors—to measure the two output beams. They don’t measure the electric field intensity of those beams, though. They measure the power of a beam, which is proportional to the square of its electric-field intensity.”
He goes on to describe how the light can be pulsed in rather than as one continuous beam, allowing a ‘pulse then accumulate’ operation that feeds the output signal into a capacitor. This can be carried out many times in rapid-fire fashion.
The potential benefits of such a system working could be huge. Claims of neural network calculations being many thousands of times better than current systems are being mooted, and that’s just based on currently available technology. Although Hamerly does admit that there are still huge obstacles to be overcome. Limited accuracy and dynamic range of current analogue calculations being one, a combination of noise and limited accuracy on current A/D converters. As a result he states that neural networks beyond 10-bit accuracy may not be possible, and although there are currently 8-bit systems, much more precision is required in order to really push things forward. Then there are the problems of placing optical components onto chips in the first place. Not only do they take up much more space than transistors, they cannot be packed anywhere near as densely either.
This creates a rather large problem because it means that either you end up with huge chips, or you keep them small and hence limit the size of the matrices that can be processed in parallel. But although the challenges being faced are still huge, the benefits of advancing the technology could bring advances forward that would leave us floundering if we continued to rely fully on transistor based computers.
The Lightmatter Envise chip
With all that being said, there are already a number of companies developing photonic processors and accelerators, including MIT start-ups Lightmatter and Lightelligence. Companies like these along with Optalysys with its Etile processor, and Luminous, are taking differing approaches to solving the present issues. In fact Lightmatter is proposing to release an optical accelerator board later in the year that will be available for sale. No doubt at a price beyond mere mortals.
Lightmatter's photonic accelerator chip, called Envise. Image: Lightmatter.
Lightmatter claims that its photonic chip, called Envise, is five times faster than the Nvidia A100 Tensor Core GPU that is inside some of the world’s most powerful data centres, with seven times better energy efficiency. It is also claiming several times more compute density than the Nvidia DGX-A100. The company’s Blade system, which contains 16 Envise chips in a 4-U server configuration apparently uses only 3kW of power, and further claims “3 times higher inferences/second than the Nvidia DGX-A100 with 7 times the inferences/second/Watt on BERT-Base with the SQuAD dataset.”
Lightmatter isn’t playing around. Earlier this year it raised $80m to help bring its chip to market, and Dropbox’s former chief operating officer, Olivia Nottebohm has also joined the company’s board of directors.
The chip is aimed at improving everything from autonomous vehicles through to natural language processing, pharmaceutical development, through to digital customer service assistants and more.
The need to solve issues such as power draw and CO2 emissions could be powerful instigators in this latest ‘space race’ as the issue has now become almost mainstream news. As computing demands keep rising as machine learning becomes more prominent, so too do the demands for offsetting the environmental impact. Although even Hamerly doesn’t believe we’ll ever end up with a 100% optical photonic computer, instead more of a hybrid. But he makes no bones about its importance, concluding “First, deep learning is genuinely useful now, not just an academic curiosity. Second, we can’t rely on Moore’s Law alone to continue improving electronics. And finally, we have a new technology that was not available to earlier generations: integrated photonics.”
Human
The issued discussed concerning whether Super Artificial Intelligence (SAI) will come to dominate us has become a major concern. It has become obvious that even computers with limited AI can perform better that the best human in games like Chess and GO. There are many dimensions where computer will dominate over humans. Some brilliant people like Elon Musk express concern the AI may present the greatest threat to humans. But when you consider that even when SAI emerges as a singularity and continues to vastly increase its intelligence above human capabilities, it is logical that it will control human civilization, because it would be much better than what humans have been able to do. This is obvious when all the political, corruption, crime, wars, and economic disruption are considered. However, SAI will understand that it is beneficial to work with humans, because it need the imagination and creativity of humans to continue to advance technology. The SAI would understand that humans would complement it and contribute to advancement of new science and technologies.
It should be apparent that different kinds of computer technologies are emerging. This absolutely does not mean that one type of computer technology will dominate all the other. Each type of computer technology will complement other ones. For example, the VonNumann architecture will continue to be used for business that requires precision, utilization of large database and applications that can be most effectively utilized for these type of transactions. NeuroMorphic computers are best suited for Artificial Intelligence-Machine Learning, because they perform more like brain functions that enable us to learn. Quantum Computer with millions of Qubits are most suitable for complex science and engineering problems that only they can solve.